Getting your Trinity Audio player ready…
|
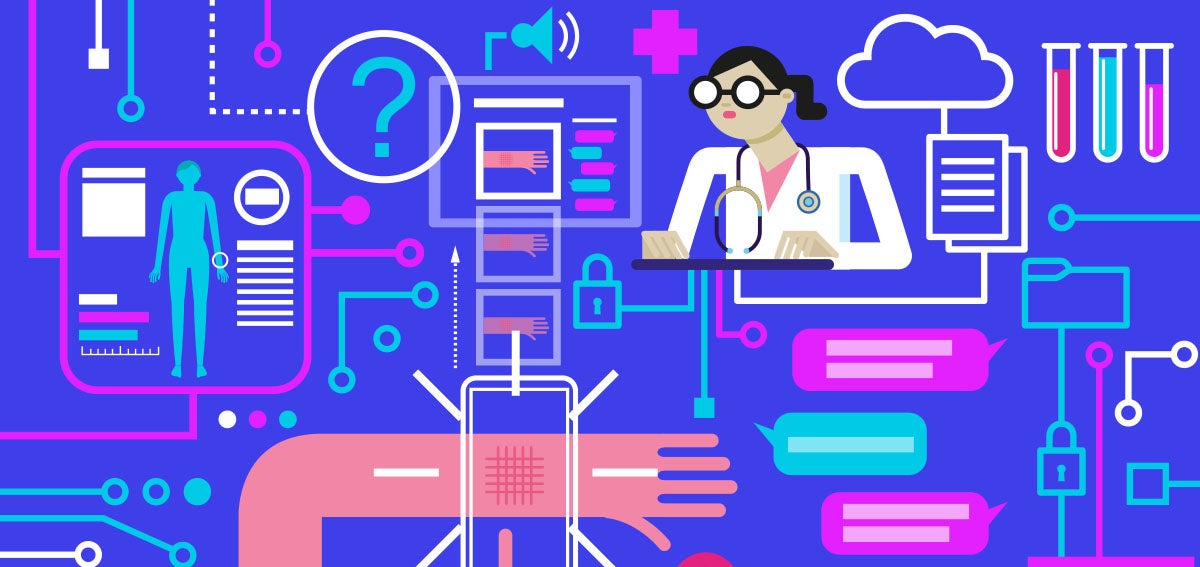
Artificial intelligence (AI) holds immense promise as a great equalizer — a technology that can be harnessed to lift up historically marginalized communities by increasing access to care, improving provider efficiency, and broadening data processing capabilities. It also poses significant risks when it comes to harming underserved populations. If AI is implemented without thoughtful consideration, it could perpetuate harmful biases, widen existing gaps in care, and create new disparities.
To learn more about how California safety-net health plans are grappling with these complex challenges and opportunities, CHCF spoke with chief health equity officers from three Medi-Cal plans serving highly diverse populations. They are Pooja Mittal, MD, of Health Net; Mohamed Jalloh, PharmD, of Partnership Health Plan; and Traco Matthews, MBA, of Kern Health Systems.
These conversations yielded insights into how safety-net health plans are thinking about leveraging AI to improve health outcomes, while ensuring it is adopted fairly and in ways that do not create unintended consequences. These experts addressed the risks of exacerbating existing health disparities and emphasized the importance of prioritizing inclusion and fairness throughout the AI life cycle, from development to deployment.
Here are key takeaways from those conversations:
Leverage interest in AI’s extraordinary data-mining capacity to help historically marginalized communities. AI can enhance care for underserved populations through its capacity to analyze vast amounts of data to surface insights, identify specific high-risk patients, and shape personalized interventions.
“I see potential for us being able to use AI to dive deep into unstructured data in the electronic health record (EHR) to look for what we need in a way that’s much more efficient,” said Mittal. Health Net’s membership base includes “every language, race, ethnicity, gender identity, and sexual orientation” across rural and urban geographies, she added.
At Partnership Health Plan, there is great enthusiasm for AI’s possibilities, Jalloh explained. “We have an amazing new chief information officer who’s been leading a lot of the activities, and they actually started an official working group to lead AI,” he said. “This is something we’re really excited about.”
Matthews outlined how Kern Health Systems is actively exploring AI applications. “Our journey towards AI is similar in many regards to health equity,” he said. “It’s just technology providing support for what you are trying to accomplish in terms of serving your members, understanding things about them, and having some automated analysis that is outside of the normal human purview. That’s been happening for a long time. We are intentionally moving in a direction where we’re embracing some things that are now considered under the umbrella of artificial intelligence,” he said.
As an example, Matthews described promising progress around applying AI to integrate member data and automate personalized outreach. “We do not currently have a customer relationship management tool,” he said, adding that the organization has “very siloed information for [its] members.” He recounted a conversation with a Kern Health colleague about how the plan could use AI to better organize and sift through member data to send automated wellness check messages, or reschedule missed appointments more efficiently.
“It’s really important that we think through some sort of equity allocations now, as we’re on the verge of huge breakthroughs and all of these wonderful wins and gains for artificial intelligence.”
—Traco Matthews, Kern Health Systems
Some plans already run programs reliant on AI, such as Start Smart for Baby, a wrap-around pregnancy program that supports moderate- or high-risk pregnant members at Centene, the parent organization of Health Net. “We’ve incorporated a machine learning algorithm into it to ensure that we’re able to focus on members who are highest risk,” said Mittal.
The algorithm is trained to flag for possible health issues — such as high blood pressure, which may indicate that the person would be a good candidate for taking low-dose aspirin during pregnancy — that the plan can then communicate to the member’s provider. “The algorithm is what really helps us go down that path,” said Mittal. The program focuses on broad past medical history as well as individual risk factors such as race, ethnicity, and language to achieve greater precision in its risk assessment.
Establish equitable infrastructure and resources. Mittal emphasized the need to familiarize people who work at managed care plans and safety-net health systems with generative AI so they can understand its potential and its pitfalls. “We’re quite early in the use of generative AI, and I think there’s a lot of caution around how to be equitable and ensure there’s no bias before we go down that path.”
In rural communities, a lack of broadband access stymies the adoption of advanced, cloud-based AI programs that require high data-processing speeds. “That doesn’t just impact people,” Matthews said. “That impacts businesses, clinics, and providers. So that is potentially a barrier to the quality of the technology that you are leveraging and therefore the quality of the artificial intelligence and technological support that you might be able to provide.”
Other challenges have more to do with finances. Clinics with limited resources may use older EHR programs that are incompatible with new technologies, or they may lack the resources to train personnel in the use of new platforms. “If you go to these clinics that are understaffed, underfunded, and have the bare minimum EHR, you can come with this new tool, but it’s not going to be helpful,” said Jalloh.
“When you introduce something new, especially something you don’t have a lot of information about, it’s easy to be scared of it.”
—Mohamed Jalloh, Partnership Health Plan
The solution lies in opening funding pathways so that under-resourced clinics and communities can have equitable access to new health care technologies. Only through targeted investments and dedicated funding streams will all facilities and communities be able to implement AI. One idea that appeals to Jalloh is for a portion of profits generated from health care AI projects to be reinvested in IT infrastructure and training support for under-resourced facilities — and the sooner, the better. “The reality is that AI is needed,” he said. “You might as well start building that infrastructure now.”
It’s going to be difficult to advance AI in these communities without some sort of equity allocations, Matthews said. Otherwise, today’s disparities will persist for years. “It’s really important that we think through that now, as we’re on the verge of huge breakthroughs and all of these wonderful wins and gains for artificial intelligence,” Matthews said.
Build trust through education about AI. Mistrust is a barrier to widespread implementation and acceptance of AI, especially in communities where historic inequities have long existed. “Trust is already lower for certain populations across the state, across the nation, and right here in Bakersfield and in Kern County,” said Matthews. Matthews, a Black man, recounted how his father elected not to get the Covid vaccine partly because Matthews’ mother had traumatizing health care experiences. “He’s like, ‘I don’t trust the health care system,’ and he attributed a lot of that to his race,” Matthews said.
“When you introduce something new, especially something you don’t have a lot of information about, then yeah, it’s easy to be scared of it,” said Jalloh. Education will be a key way to push past fears to create acceptance and understanding, he said.
Mittal agrees that education about AI is crucial — especially as technology evolves. “A lot of it is people not understanding that there are different types of AI, that machine learning is different from generative AI,” Mittal said. “We have to start building that into how we’re teaching because until people get comfortable with how it works, we’re going to be fairly limited in how we can use it.”
Matthews emphasized the power of trusted messengers. “Trust is restored heart to heart, human to human,” he said. “People will be reticent to buy into it without a lot of trust building, bridge building, assurances, and follow through. You don’t need technical expertise to have a heart-to-heart conversation.”
Prioritize diversity and transparency. Prioritizing diversity in AI means including developers representing an array of racial and ethnic backgrounds, partnering with community organizations, compensating community members, and centering the perspectives of people with lived experience.
“We need to make sure that whoever is creating these new models is implementing diverse patient and public involvement,” said Mittal. They will need to ensure that data inputs reflect a wide variety of demographic variables, including race, ethnicity, language, gender, and geographic location, she said.
“Make sure you have diverse people who are likely going to use it and that those people are helping to contribute to the models,” Jalloh said. “So, instead of only looking at data from a certain patient group, you validate that information against multiple groups. Then you can make sure that it’s not biased.”
Matthews added that broad representation requires that people with lived experience be involved from the start — including as members of governing bodies. “As you’re developing something, bring in folks who you think will want to use it,” he said. “That will help you to not forget or marginalize those perspectives because you’re intentionally incorporating them into the process from the beginning.”
Authors & Contributors
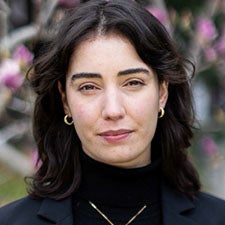
Robin Buller
Robin Buller is an Oakland-based writer, researcher, and editor. She has reported on harm reduction, maternal health, migration, housing, and policing for The Guardian, The Oaklandside, and other publications.
Originally from Canada, she holds a doctorate in history from UNC Chapel Hill and has lived in California since 2018.
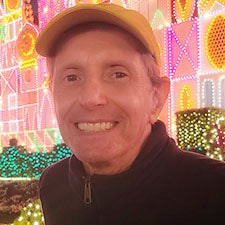
Dan Sipple
Dan Sipple is a Los Angeles illustrator/designer with the ability to solve creative problems with unique visual solutions.
A partial client list includes: Merck, McDonald’s, Sony, Seagram’s, Absolut, Proctor and Gamble, the Boston Globe, Better Homes and Gardens, National Geographic Kids, Astra-Zeneca, Brach’s, Hasbro, Intel, Fisher-Price, Chronicle Books and as many other clients large and small.